Welcome to Protein Scaffold Filler Tool.
Generate Complete Protein Sequence Fill Gaps Of Scaffold Train Model and Predict on New Scaffold
Features
Our Protein Scaffold Filler employs machine learning, deep learning and generative AI techniques to effortlessly fill gaps in protein scaffolds, creating complete sequences. Currently, we have trained the model on Mabcampath data. You can try to fill the simulated scaffold of these data or train on new scaffold on our system and fill the scaffold.
How it Works?
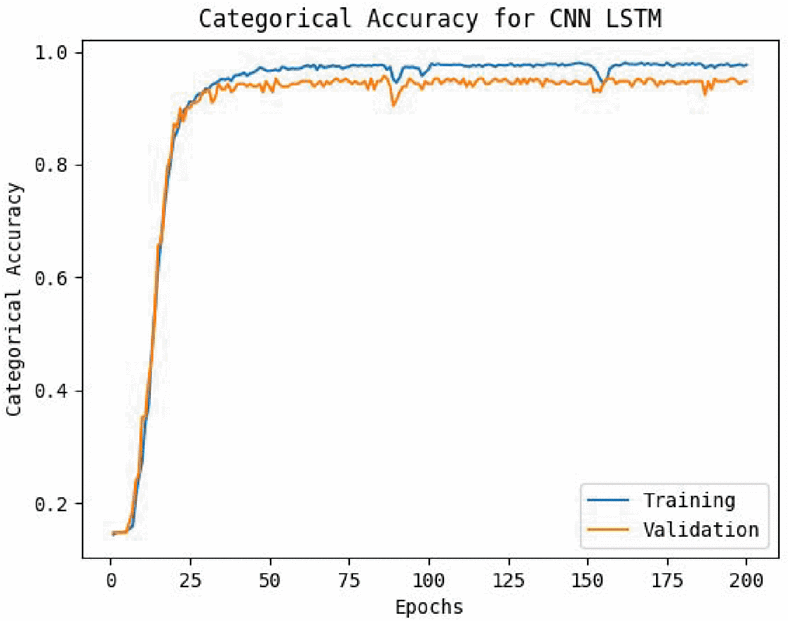
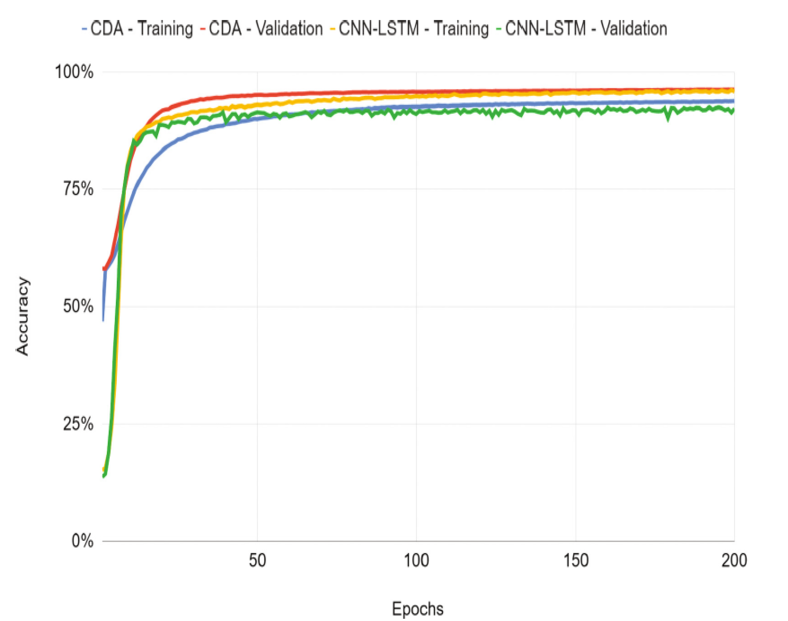
Contributors
This work is supported by the National Science Foundation of the United States under Award 2307571, 2307572 and 2307573. We thank everyone who has helped us directly or indirectly.
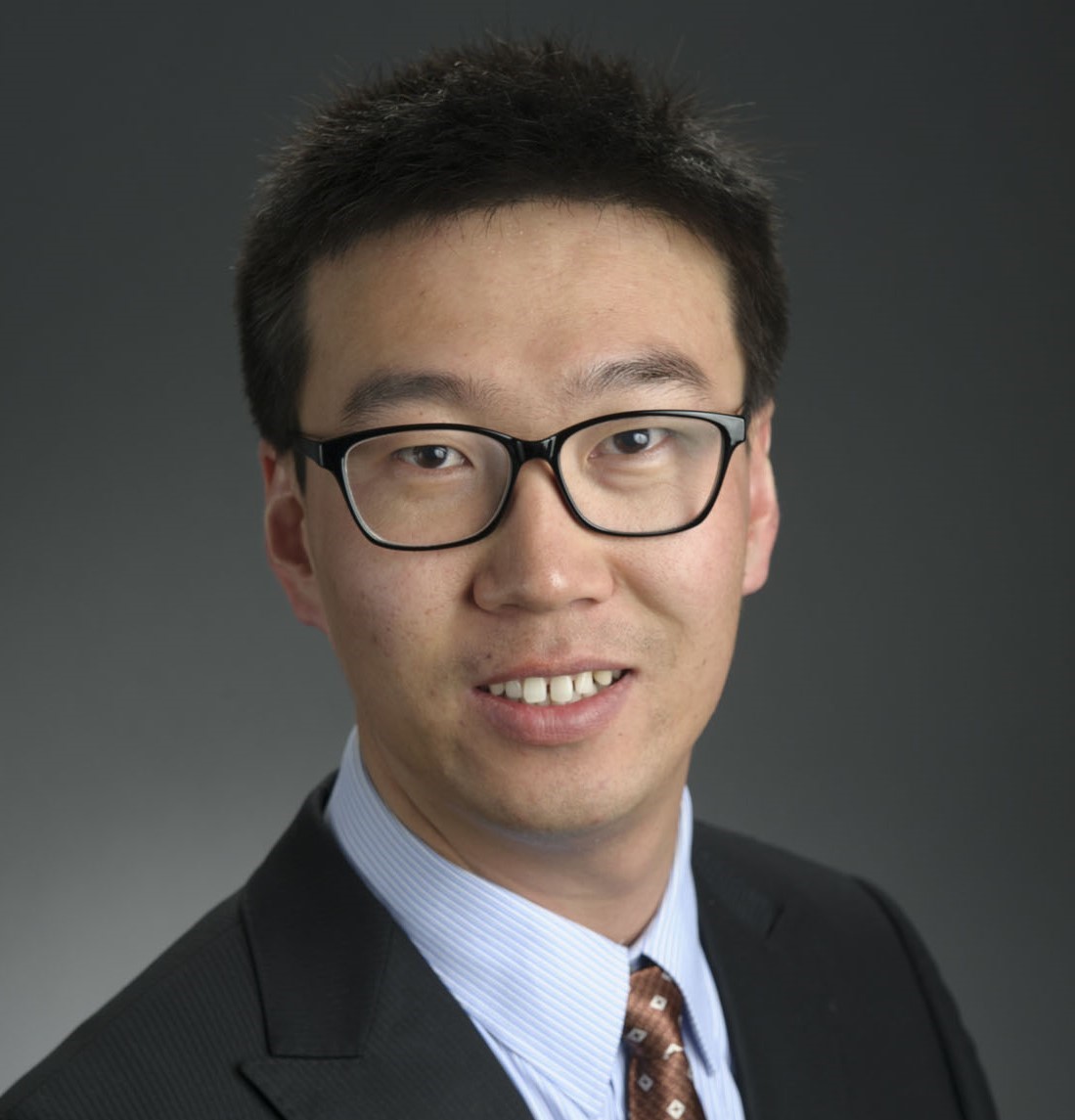
Dr. Letu Qingge
Dr. Letu Qingge, Ph.D, is an assistant professor in the Department of Computer Science at North Carolina A&T State University, NC. He received his Ph.D degree in Computer Science from Montana State University, MT, USA. His research interests include algorithms, machine learning, deep learning, cloud computing, combinatorial optimization, bioinformatics and computational biology, computer vision, transportation network, and neural network control. His research has been funded by NSF and NIH.
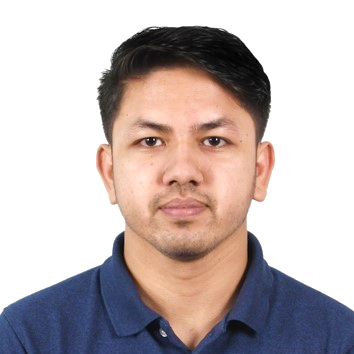
Mr. Kushal Badal
Kushal Badal is currently a Master student with a strong academic record and a passion for AI and data science. He gained practical experience in data analysis, machine learning, and research, complemented by leadership roles and a commitment to continuous learning. His goal is to leverage his technical skills and enthusiasm for problem-solving to make meaningful contributions in the field of AI.
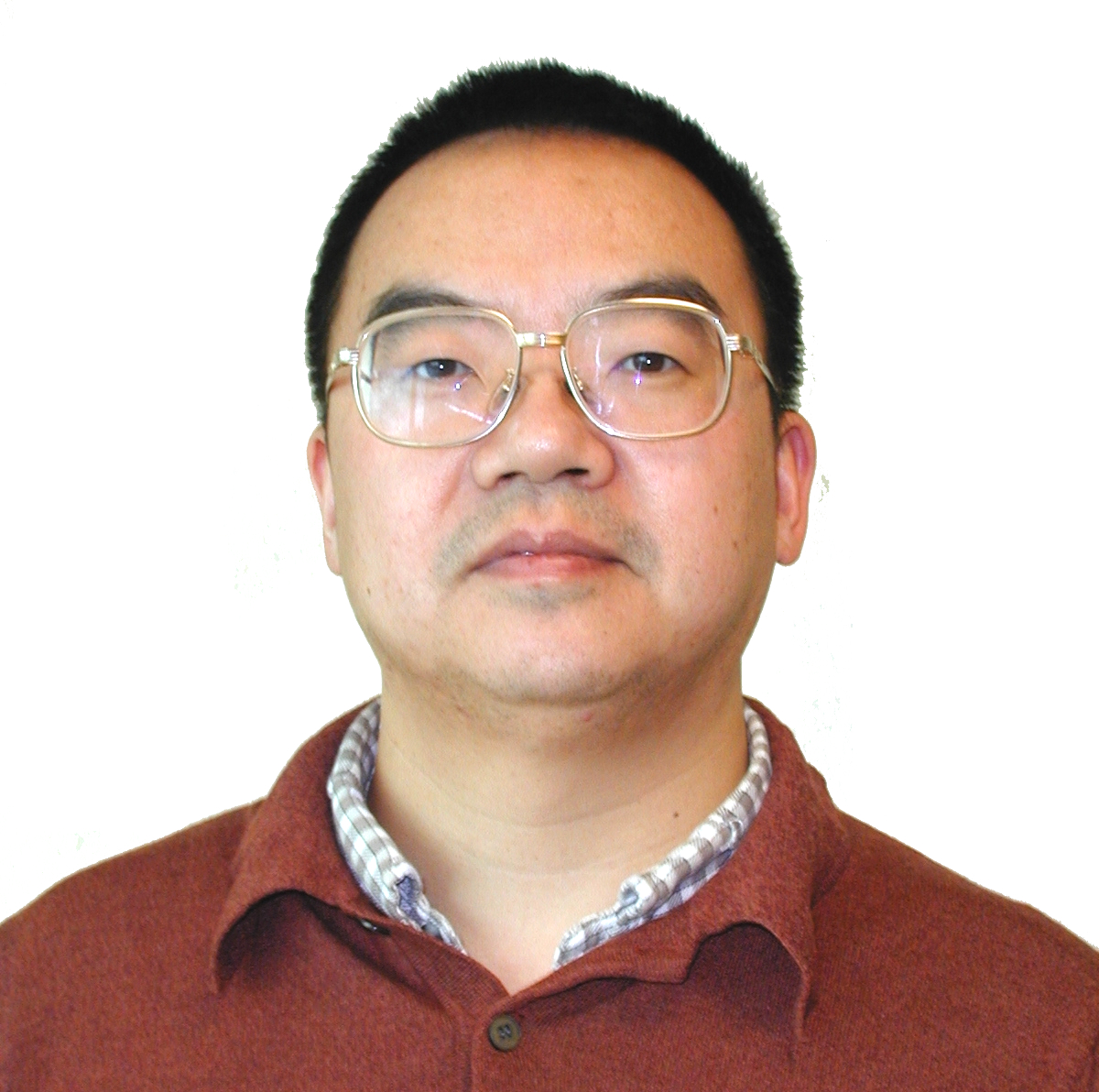
Dr. Binhai Zhu
Dr. Binhai Zhu obtained his PhD in computer science at McGill University in 1994. Following a 2-year post-doc at Los Alamos National Laboratory, he has been teaching at City University of Hong Kong and Montana State University since Aug, 1996. He is currently a professor at Gianforte School of Computing at Montana State University. His research interests are Algorithms, Computational Biology, Computational Geometry, and Combinatorial Optimization. He has published over 220 papers in these areas. He is currently on the editorial board of Acta Informatica and Algorithms.
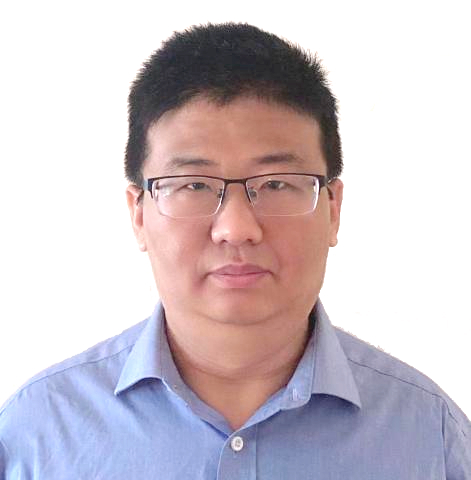
Dr. Xiaowen Liu
Dr. Liu received his bachelor’s (1996) and master’s (2004) degrees in computer science from Shandong University, China and obtained his Ph.D. degree (2008) in computer science from City University of Hong Kong, Hong Kong. After 4-year postdoc training at the University of Western Ontario, the University of Waterloo, and the University of California, San Diego, Dr. Liu took positions as an Assistant Professor and Associate Professor at the Department of BioHealth Informatics, Indiana University-Purdue University Indianapolis from 2012 to 2021. Dr. Liu is currently a professor of bioinformatics in the Division of Biomedical Informatics and Genomics, John W. Deming Department of Medicine, Tulane University School of Medicine. Dr. Liu’s research focuses on computational proteomics, especially mass spectrometry-based top-down proteomics, which can identify various proteoforms with alterations in biological samples. Dr. Liu’s lab is supported by several NIH grants, and his work has resulted in more than 80 peer-reviewed journal papers.